Research Reports
A Study on the Agricultural Production Survey Methods Using Drones
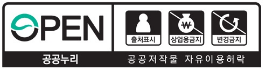
-
AuthorGouk, Seungyong
-
Publication Date2023.02.17
-
Original
Purpose of Research
○The aim of this study is to verify if agricultural production surveys utilizing drones can have sufficient reliability and to present various uses of drones and related standards. The study examines if crops can be identified in images acquired by drones and what quality levels of drone images enable the identification of crops. We present ways to use drones to acquire images of proper quality; and examine if plant density can be found by utilizing drone images and if this process can be automated. Based on the research results, this study presents methods of using drones in agricultural production surveys.
Research Method
○A blind experiment was conducted to compute proper GSDs for crop identification. For six months from March to August, when diverse crops are grown, images of research target crops were taken each month. The six GSDs of each crop’s images were as follows: 5, 4, 3, 2, 1, and 0.5cm. In our experiment, we asked experts who can identify crops what GSD levels of the images enable crop identification, and asked them to identify the crops. Then we comprehensively analyzed the GSD levels that enable crop identification according to the experts and the accuracy of their identification. Although experts participate in the blind experiment, the method has a similar aspect to machine learning. Therefore, we utilized the confusion matrix analysis widely used in machine learning.
○A YOLO(You Only Look Once) model was applied to calculate the number of plants grown using artificial intelligence. The model utilizes a CNN(Convolutional Neural Network) in the pre-training process. To correct the deep learning individual identification model’s misclassification, we used the identification of each crop’s center and the distance analysis between the centers. Also, various statistical techniques were applied to enhance the accuracy of the number of plants grown.
(For more information, please refer to the report.)
- Next
- Reforming the Roles of the Agricultural Holding Registration System: Challenges and Suggestions
- Prev
- A Study on the Future Scenarios of Korean Agriculture in 2040